Integrating discipline specific AI into your teaching
In this blog Dr Kathy Charles, Executive Dean of Learning & Teaching at NTU explores how AI might be incorporated into your teaching.
Please note, the views expressed in this article are the opinion of the author. For NTU policy and guidance in this area please see:
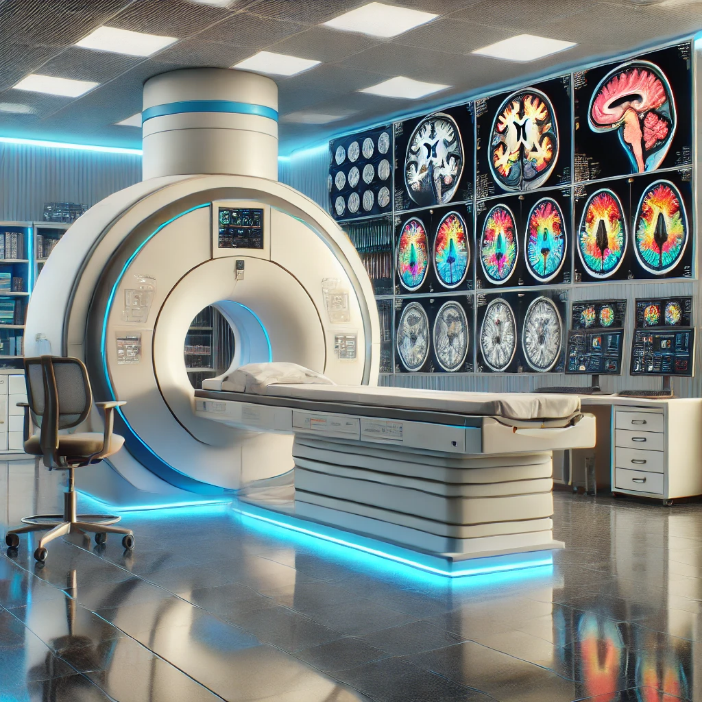
Earlier this year, I completed the Oxford Artificial Intelligence Programme, a six-week online course that explored AI from a wide range of perspectives, with weekly assessments to deepen understanding. I found the program both challenging and rewarding. It pushed me to look beyond the sometimes overegged promise of AI revolutionising teaching combined with the anxieties surrounding academic integrity and AI. The diverse backgrounds of my fellow students, who varied greatly in their knowledge and use of AI, enriched our discussions and provided fresh insights that complemented the course content.
Much has already been written about how AI can enhance and support teaching and learning, so I won’t dwell on that. The debate around AI's role in cheating at assessments and the countermeasures AI might offer has also been thoroughly explored. Instead, I want to focus on something less often discussed: how we can integrate AI into our specific subject disciplines. Drawing from my academic background in psychology, I’ll explore how AI might be incorporated into the subject itself.
Incorporating AI into psychology demonstrates its transformative potential
If I were teaching psychology this year, I would engage my students in discussions about how AI could impact psychology as both an academic discipline and a professional practice. A compelling example is neuroimaging, a subject that can be incorporated into multiple areas of psychology and offers a clear illustration of AI’s transformative potential.
AI can revolutionise neuroimaging by enhancing the diagnosis, treatment, and understanding of neurological disorders. Advanced machine learning algorithms allow AI to process and analyse the vast amounts of data generated by neuroimaging techniques such as MRI, fMRI, and PET scans. This capability is changing how researchers and clinicians interpret brain images, leading to more accurate diagnoses and paving the way for personalised medicine.
AI algorithms can identify patterns and anomalies that might not be immediately apparent to the human eye.
Traditional methods of analysing neuroimaging data rely heavily on expert interpretation, a process that can be both time-consuming and prone to error. AI, particularly deep learning algorithms, can automate this process by identifying patterns and anomalies in brain images that might not be immediately apparent to the human eye. For a broader context, the paper by Litjens et al. (2017) provides an excellent overview of AI in medical imaging as it stood in 2017, while Thirion (2024) offers a more recent focus on neuroimaging specifically.
AI is also accelerating neuroscience research by enabling the analysis of increasingly large datasets. AI can detect subtle patterns in these datasets, leading to new insights into brain function and structure. For instance, AI has been used to analyse functional connectivity in the brain, deepening understanding of how different brain regions communicate. This has significant implications for understanding complex neurological conditions like schizophrenia and autism.
Looking ahead, AI holds the promise of advancing personalised medicine. By analysing an individual’s neuroimaging data alongside other health information, AI could help develop customised treatment plans tailored to the patient’s unique brain structure and function. This approach could revolutionise the treatment of conditions like epilepsy, where the effectiveness of treatments can vary widely among patients.
It can serve as a gateway for students to understand reinforcement learning in algorithms, helping them grasp the broader workings of AI
Incorporating AI into psychology not only enhances understanding of the subject but also provides a platform to explore the ethics and limitations of AI. By discussing how these technologies might evolve, how far they could be taken, and how to design research to test these ideas, students can engage more deeply with the subject matter. This approach also facilitates discussions on the data needed to drive AI, the sources of these data, and the challenges that arise when AI-driven decisions go wrong. It can serve as a gateway for students to understand reinforcement learning in algorithms, helping them grasp the broader workings of AI. Exploring AI in this way becomes a thought-provoking process for exploring human judgment, empathy, and critical thinking—key components in psychology and many other subjects.
In my view, psychology is an excellent discipline for exploring and contextualising AI. From sentiment analysis to brain injury recovery, to risk assessment and big data analysis, AI touches every corner of the subject. However, I believe AI can be incorporated into many other disciplines as well. With the assistance of CoPilot, I have also explored how AI might be integrated into fields far removed from my own
CoPilot can also assist in considering the ethical and social implications of using AI in different contexts.
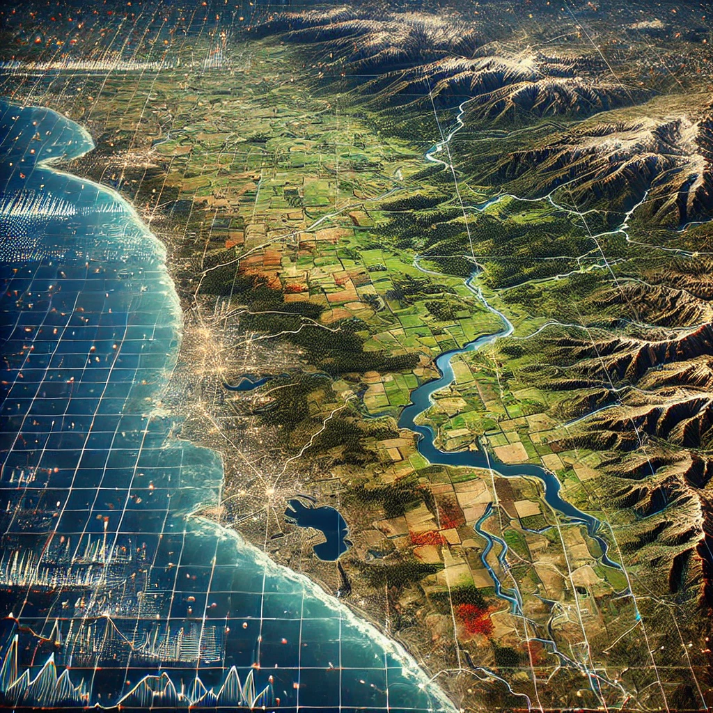
Take geography, for example. AI can be used for object detection and classification in remote sensing data, identifying specific types of vegetation, buildings, or vehicles in satellite images. This capability greatly enhances the accuracy and speed of environmental monitoring, urban planning, and resource management. AI can also automate the detection of landscape changes over time, such as deforestation, glacier retreat, or urban expansion—crucial for monitoring environmental changes and managing natural resources. With a bit more prompting, CoPilot can also assist in considering the ethical and social implications of using AI in this context, leading to discussions as wide ranging as those I’ve outlined with neuroimaging.
No discipline remains untouched by AI
If you’ve grown weary of the constant utopian and dystopian visions of generative AI in teaching and learning, I encourage you to take a step back and explore the wider applications of AI within your own subject. No discipline remains untouched by AI, and no subject is immune to its social and ethical considerations. Introducing students to AI through the familiarity of their subject choice can give it greater meaning and relevance.
Perhaps you’re already incorporating discipline related AI into your teaching and have valuable examples to share with your colleagues at one of our TILT events. Please volunteer if that’s the case! If you’d like support or advice on using AI in your teaching, please get in touch with us in CADQ.
Author Information:
Dr Kathy Charles is Executive Dean of Learning & Teaching and head of the Centre for Academic Development & Quality at NTU.
More from CADQ Blogs
NTU in Mansfield - Widening access to Higher Education by connecting with local industry
Tom Withers Partnerships Senior Standards & Quality Officer and Stuart Parkin Educational Developer share how NTU in Mansfield is widening access to higher education.
TILT - It's all about Community
Laura Stinson, Senior Academic Practice Developer (TILT Lead), talks TILT® (Trent Institute of Learning & Teaching) and how it supports colleagues' professional development at NTU
SCALE-UP: 10 years on
Dr Ellen Nicholls and Anita Love reflect on a decade of SCALE-UP at NTU, and what the future looks like for this pedagogy.