Group
Cognitive Computing and Brain Informatics Research Group (CCBI)
Unit(s) of assessment: Computer Science and Informatics
School: School of Science and Technology
Welcome to Cognitive Computing and Brain Informatics
The Group activity is centred around brain research, mainly brain computer interfacing (BCI) including EEG hyperscanning, and body sensor networking (BCI) for body and brain abnormality identification. Recently our main interest has been on seizure identification and stimulation of brain epileptogenic networks. This is through developing new adaptive and cooperative signal processing, model and data driven AI, and single- or multi-channel, multi-dimensional, and multimodal biomedical signal decomposition. The Group activity is centred around brain research, mainly brain computer interfacing (BCI) including EEG hyperscanning, and body sensor networking (BCI) for body and brain abnormality identification. Recently our main interest has been on seizure identification and stimulation of brain epileptogenic networks. This is through developing new adaptive and cooperative signal processing, model and data driven AI, and single- or multi-channel, multi-dimensional, and multimodal biomedical signal decomposition.
Our Focus
- Human-centred AI for Cognitive Computing and Brain Informatics
- Brain research (looking at functional, personal, behavioural, psychological and physiological disorders)
- Brain functional data and signal (EEG, MEG, fMRI, joint EEG-fMRI) processing, understanding, and machine learning (seizure, dementia, autism, mental fatigue, sleep, pain, depression)
- Brain-computer interfacing (BCI), brain EEG hyperscanning, mental and physical rehabilitation, and rehabilitative assessment
- Analysis, processing, and learning of human related data
- Multimodal data analysis and learning (EEG-fMRI, audio-video, medical data)
- Body sensor networking
Signal processing, machine learning, and sensor networks are the major tools in biomedical data (signal, image, and patient peripheral information) understanding, recognition, and classification. In CCBI the major research focus is on developing ground breaking approaches to Human-centred AI, Brain research (looking at functional, personal, behavioural, psychological and physiological disorders), brain functional data and signal (EEG, MEG, fMRI, joint EEG-fMRI) processing, understanding, and machine learning (brain responses to various stimuli, seizure, dementia, autism, mental fatigue, sleep, pain, depression, mild cognitive impairment – MCI and Alzheimer,) brain-computer interfacing (BCI), brain EEG hyperscanning, mental and physical rehabilitation, and rehabilitative assessment, analysis, processing, and learning of human related data, multimodal data analysis and learning (EEG-fMRI, audio-video, medical data), and body sensor networking.
Core services we offer include:
- Developing tools and algorithms for identification, classification, and scoring of generative and degenerative brain states, diseases and abnormalities.
- Assisting with multi-modal real-time medical data analysis for monitoring, diagnosis, prediction of alarming states, and decision making.
- Developing tools for Brain-computer interfacing particularly for rehabilitation.
- Developing model-driven (for data fusion), data driven (for deep learning), and diffusion techniques (for cooperative learning) for AI and machine learning.
- Speech processing for source separation, human identification, speech recognition, and speaker identification.
- Medical image enhancement, fusion, anomaly detection, and diagnosis.
- Advanced signal processing including processing of biomedical signals, speech, music, and medical images.
Our team
Professor Saeid Sanei
Email: saeid.sanei@ntu.ac.uk
Dr Isibor Kennedy Ihianle
Senior Lecturer
Computer Science
Alicia Falcon Caro
Academic Associate
Computer Science
Mufti Mahmud
Associate Professor
Department of Computer Science; Computing and Informatics Research Centre; Medical Technologies Innovation Facility
Kayode Owa
Senior Lecturer
Computer Science
Ahmet Omurtag
Senior Lecturer
School of Science & Technology
Alex Sumich
Professor
School of Social Sciences
Joao Filipe Ferreira
Senior Lecturer
School of Science and Technology – Computer Science
Jason Smith
Senior Lecturer
Physics and Mathematics
Sensor based gait recognition
New wearable sensors can circumvent the limitations of video-based gait recognition often used for rehabilitative assessment.
Body sensoring network
The information from wearable sensors can reveal physical, behavioural, and mental abnormalities and be integrated within an IoT system.
Intracranial brain stimulation
By processing the information from multichannel electrocorticogram mat the seizure networks in deep brain can be identified and localised.
Localization of brain event potentials for audio stimulation
Many human mental states and abnormalities can be identified by evaluating the brain responses to audio, visual, and haptic stimulations as the basis for brain-computer interfacing.
Selected Partners and Collaborators
The number of partners addresses NTU’s research strategy by focusing research and is external facing, with the potential to provide high quality outputs. The PhD experience is significantly enhanced by providing real world, high impact research projects to potential students.




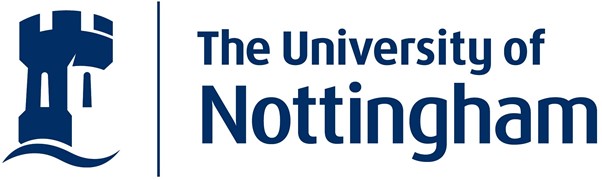

- Electrical Engineering, Imperial College London
- Neuroscience, King’s College London
- Electronics, Royal Holloway University London
- Electrical Engineering, Edinburgh University, Edinburgh, Scotland
- Computer Science, Essex University, Essex
- RIKEN Brain Institute, Japan
- University of Toronto, Canada
- Electrical and Electronic Engineering, National University of Singapore, Singapore
- Computer Science, Shiraz University, Iran
- Electrical Engineering, Ilam University, Iran
Group Publications
Most recent selected ones:
1. S. Shirani, A. Valentin, G. Alarcon, F. Kazi, and S. Sanei, “Separating inhibitory and excitatory responses of epileptic brain to single-pulse electrical stimulation,” International Journal of Neural Systems, 33:2, 2023, 2350008 (9 pages), DoI. 10.1142/S0129065723500089.
2. A. Mobaien, R. Boostani, M. Mohammadi, and S. Sanei, “ERP detection based on smoothness priors” IEEE Transactions on Biomedical Engineering, vol. 70, no. 3, 867-876, 2023, Doi. 10.1109/TBME.2022.3204506.
3. A. Zandbagleh, S. Mirzakuchaki, M. R. Daliri, P. Premkumar, Luis Carretie and S. Sanei, “Tensor factorization approach for ERP-based assessment of schizotypy in a novel auditory oddball task on perceived family stress,” Journal of Neural Engineering, 19 066028, 2022 10.1088/1741-2552/aca69f.
4. H. Azami, S. Sanei, and T. K. Rajji, “Ensemble entropy: A low bias approach for data analysis”, Journal of Knowledge-Based Systems, online: vol. 28 Nov. 2022, 109876, Doi. 10.1016/j.knosys.2022.109876. (IF=8.038).
5. N. Goshtasbi, R. Boostani, and S. Sanei, “SleepFCN: A fully convolutional deep learning framework for sleep stage classification using single-channel electroencephalograms,” IEEE Transactions on Neural Systems and Rehabilitation Engineering, vol. 30, pp. 2088-2096, 2022, 10.1109/tnsre.2022.3192988
6. B. Abdi-Sargezeh, A. Valentin, G. Alarcon, D. Martin-Lopez, and S. Sanei, “Sparse common feature analysis for detection of interictal epileptiform discharges from scalp EEG using concurrent intracranial-scalp recordings,” IEEE Access, vol. 10, pp. 49892-49904, 2022 doi. 10.1109/ACCESS.2022.3167433.
7. S. Hashemipour, R. Boostani, and S. Sanei, “Continuous scoring of depression from EEG signals via a hybrid of convolutional neural networks, IEEE Transactions on Neural Systems and Rehabilitation, vol. 30, 176-183, 2022, doi. 10.1109/TNSRE.2022.3143162.
8. A. Zandbagleh, S. Mirzakuchaki, M. R. Daliri, P. Premkumar, and S. Sanei, “Schizotypy assessment via evaluation of brain connectivity,” International Journal of Neural Systems, Vol. 32, No. 4 (2022) 2250013 (17 pages) [IF=6.7]
9. V. Vahidpour, A. Rastegarnia, A. Khalili, W. M. Bazzi, and S. Sanei, “Energy-efficient diffusion Kalman filtering for multi-agent networks in IoT" IEEE Internet of Things Journal, vol. 9, no. 8, 6277-6287, 2022. Doi. 10.1109/JIOT.2021.3111593 (IF=9.936).
10. B. Abdi-Sargezeh, A. Valentin, G. Alarcon, D. Martin-Lopez, and S. Sanei, “Higher-order tensor decomposition based scalp-to-intracranial EEG projection for detection of interictal epileptiform discharges,” Journal of Neural Engineering 18 (6), 066039, 2021, doi. 10.1088/1741-2552/ac3cc4.
11. M. Mahmoodi, B. Makkiabadi, M. Mahmoudi, and S. Sanei, “A new method for accurate detection of movement intention from single channel EEG for online BCI,” Computer Methods and Programs in Biomedicine Update, Available online 20 August 2021, 100027, 10.1016/j.cmpbup.2021.100027, [IF=5.428].
12. S. Afshar, R. Boostani, and S. Sanei, “A combinatorial deep learning structure for precise depth of anesthesia estimation from EEG Signals,” IEEE Journal of Biomedical and Health Informatics, vol. 25, issue 9, pp. 3408-3415, Doi, 10.1109/JBHI.2021.3068481, Apr. 26, 2021 [IF=5.18].
13. B. Abdi-Sargezeh, A. Valentin, G. Alarcon, and S. Sanei, “Incorporating uncertainty in data labeling into automatic detection of interictal epileptiform discharges from concurrent scalp EEG via multi-way analysis,” International Journal of Neural Systems (IJNS), Vol. 31, Issue 08, Article No. 2150019, Year 2021, 10.1142/S0129065721500192. [IF=6.7].
14. D. Jarchi, J. Kaler, and S. Sanei, “Lameness detection in cows using hierarchical deep learning and synchrosqueezed wavelet transform,” IEEE Sensors Journal, vol. 21, Issue: 7, pp. 9349-9358, Apr. 2021.
15. D. Jarchi, J. Andreu-Perez, M. Kiani, O. Vyšata, J. Kunchynka, A. Procházka, and S. Sanei, “Recognition of patient groups with sleep related disorder using bio-signal processing and deep learning,” Sensors, 20(9) 2594, 2020.
16. H. Giv, A. Khalili, A. Rastegarnia, and S. Sanei, “A robust adaptive estimation algorithm for Hamiltonian sensor networks,” IEEE Control Systems Letters 5 (4), 1243-1248, 2020.
17. V. Vahidpour, A. Khalili, A. Rastegarnia, W. Bazzi, and S. Sanei, “Variants of partial update augmented CLMS algorithm and their performance analysis,” IEEE Transactions on Signal Processing, vol. 68, no. 1, pp. 3146-3157, 2020 (doi: 10.1109/TSP.2020.2993938).
18. M. Latifi, A. Khalili, A. Rastegarnia, and S. Sanei, “A robust scalable demand-side management based on diffusion-ADMM strategy for smart grid,” IEEE Internet of Things (IoT) Journal, vol. 7, no. 4, pp. 3363-3377, DOI. 10.1109/JIOT.2020.2968539, 2020 (IF=9.52).
19. M. Latifi, A. Khalili, A. Rastegarnia, W. M. Bazzi, and S. Sanei, “A self-governed online energy management and trading for smart micro/nano-grids,” IEEE Transactions on Industrial Electronics, vol. 67, issue 1, pp. 7484-7498, 2020, 10.1109/TIE.2019.2945280. (IF = 7.5).
20. A. Prochazka, H. Charvatova, O. Vysata, D. Jarchi, and S. Sanei, “Discrimination of cycling patterns using accelerometric data and deep learning techniques", Journal of Neural Computing and Applications, 33:7603–7613, 2020. IF= 4.774 (Q1), DOI: 10.1007/s00521-020-05504-3. (IF = 4.77)
21. H. Azami, S. E. Arnold, S. Sanei, Z. Chang, G. Sapiro, J. Escudero, and A. S. Gupta, “Multiscale fluctuation-based dispersion entropy and its applications to neurological diseases,” IEEE Access, vol. 7, no. 1, pp. 68718-68733, Print ISSN: 2169-3536, 2019, DoI: 10.1109/ACCESS.2019.2918560.
22. A. Rastegarnia, P. Malekian, A. Khalili, W. M. Bazzi, and S. Sanei, “Tracking analysis of minimum kernel risk-sensitive loss algorithm under general non-Gaussian noise,” IEEE Transactions on Circuits and Systems II, Vol 66, no. 7, pp. 1262-1266, 2019. DoI: 10.1109/TCSII.2018.2874969
23. V. Vahidpour, A. Rastegarnia, A. Khalili, and S. Sanei, “Partial diffusion Kalman filtering for distributed state estimation in multiagent networks,” IEEE Transactions on Neural Networks and Learning Systems, vol. 30, no. 12, pp. 3839 – 3846, 2019, DoI 10.1109/TNNLS.2019.2899052 (IF=11.69).
24. A. Akbari, M. Trocan, S. Sanei, and B. Granado, “Joint sparse learning with nonlocal and local image priors for image error concealment," IEEE Transactions on Circuits and Systems for Video Technology, vol. 30, no. 8, pp. 2559 – 2574, 2019.
25. M. Latifi, A. Rastegarnia, A. Khalili, and S. Sanei, “Agent-based decentralized optimal charging strategy for plug-in electric vehicles” IEEE Transactions on Industrial Electronics, vol. 66, no. 5, pp. 3668-3680, 2019 DoI. 10.1109/TIE.2018.2853609 (IF = 7.5).
26. M. Latifi, A. Khalili, A. Rastegarnia, and S. Sanei, “A Bayesian real-time electric vehicle charging strategy for mitigating renewable energy fluctuations, IEEE Transactions on Industrial Informatics, vol. 15, no. 5, pp. 2555-2568, DoI. 10.1109/TII.2018.2866267, May 2019 (IF= 7.38).
27. A. Antoniades, L. Spyrou, D. Martin-Lopez, A. Valentin, G. Alarcon, S. Sanei, and C. Cheong Took, “Deep neural architectures for mapping scalp to intracranial EEG,” International Journal of Neural Systems, 28(8):1850009, 2018, DOI. 10.1142/S0129065718500090, online (IF=7).
28. S. Monajemi, S. Sanei, and S.-H. Ong, “Information reliability in complex multitask networks,” Elsevier Journal of Future Generation Computer Systems, Special Issue on Measurements and Security of Complex Networks and Systems, vol. 83, pp. 485-495, 2018. Doi. 10.1016/j.future. 2017.07.023 (IF=3.9).
29. D. Jarchi, J. Pope, T. K. M. Lee, L. Tamjidi, and S. Sanei, “A review on accelerometry based gait analysis and emerging clinical applications,” IEEE Reviews in Biomedical Engineering, vol. 11, issue 1, pp. 177-194, 2018, DOI. 10.1109/RBME.2018.2807182.